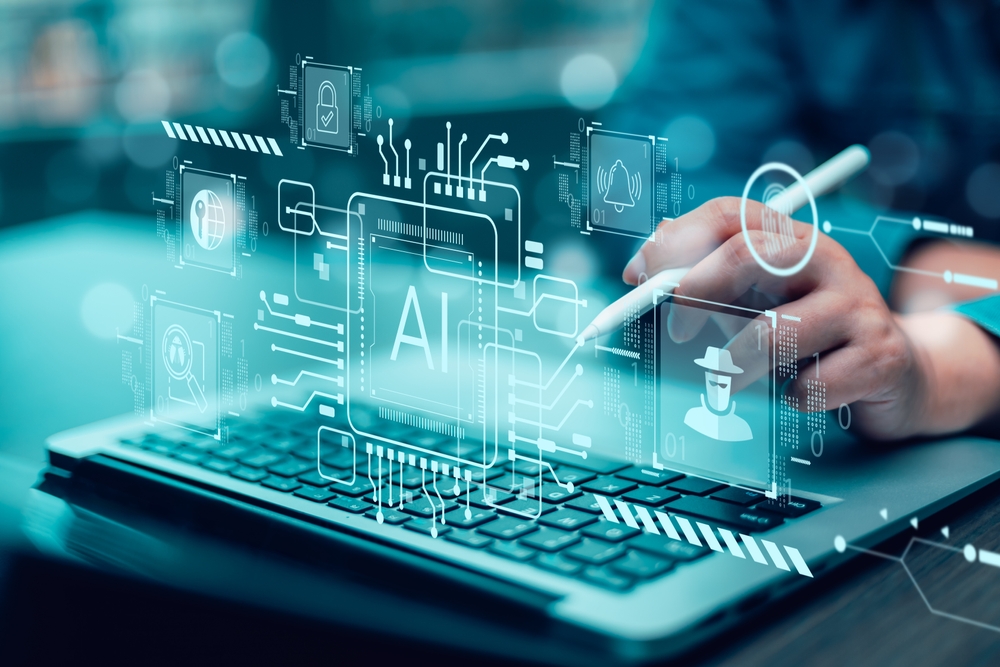
Unlocking AI in the Lab: Your 2025 Roadmap to Data Readiness & Scalable Innovation
Artificial intelligence (AI) is transforming life science research and development (R&D), enabling scientists to push new boundaries, develop personalized treatments, and tackle complex biological challenges faster and on a larger scale than ever before. As AI continues to reshape the R&D landscape, labs need to be ready to make the most of its potential through AI data readiness. This is key to ensuring smooth integration, helping researchers make better decisions, use data more effectively, and accelerate scientific breakthroughs. In blog series, we we’ll walk you through a clear roadmap to achieve AI data readiness in R&D labs, setting the stage for meaningful business transformations through 2025 and beyond.
AI/ML is not just about technology
AI data readiness in the R&D lab goes beyond technology—it’s about cultivating a work culture of collaboration, ensuring data quality, and promoting continuous learning. By developing a robust data infrastructure, strategically integrating AI and other advanced tools, and overcoming scientific challenges, labs can fully unlock AI’s potential to accelerate the research and development process.
The success of an AI enablement program in R&D depends on how well the lab’s data infrastructure, processes, and practices support AI/ML technologies. That means ensuring the data is accessible, clean, well-organized, and formatted correctly for smooth integration with AI/ML algorithms. Achieving AI data readiness is crucial for gaining actionable insights and ensuring the accuracy and efficiency of AI-driven applications. Even the most advanced algorithms can fall short without the right data in the right format.
Being AI/ML-ready isn’t just about having the right technology; it’s about having the right data, skilled people, and efficient processes to make it work effectively. Success comes from aligning technology with strong infrastructure, expertise, and strategies that foster iterative development and continuous improvement, ultimately driving meaningful results.
“Very often I am asked, ‘Which tool should I use?’ This is not the first question. Really, the first question is, what are the problems that you have in your organization… It really starts from the pain point.”– Bernard Marr, The Rise Of AI Scientists: Is Agentic AI The Future Of R&D1
What is the essential role of LIMS in the AI-powered lab?
In an AI-powered lab, a Laboratory Information Management System (LIMS) is essential for organizing and managing data, forming the foundation for the successful implementation of AI/ML models. LIMS ensures that data is stored in a clean, accessible, and standardized format, making it ready for analysis by AI systems. By tracking data provenance and maintaining stringent quality control, LIMS guarantees the accuracy of the data, allowing AI algorithms to generate reliable predictions and insights.
AI-enabled LIMS is built to integrate seamlessly with AI tools, automating data pipelines and providing real-time access to data for model training and result generation. This integration enables advanced analytics and decision support, helping labs predict outcomes, identify trends, and make faster, data-driven decisions. Together with LIMS, AI can automate tasks like sample tracking, inventory management, and equipment maintenance, greatly enhancing lab efficiency.
What is AI/ML?
AI (Artificial Intelligence) and ML (Machine Learning) in the R&D lab refer to applying advanced algorithms and computational models to enhance and accelerate research processes. These technologies enable labs to process large volumes of data, uncover patterns, and make predictions that would otherwise be difficult or time-consuming for researchers to achieve.
- AI (Artificial Intelligence) is the field of computer science focused on creating systems that can perform tasks that typically require human intelligence. These tasks include reasoning, learning, problem-solving, perception, and language understanding. AI systems aim to simulate human cognitive processes to handle complex, dynamic tasks.
- ML (Machine Learning) is a branch of AI that centers on developing algorithms that allow computers to learn from and make predictions or decisions based on data. Unlike traditional programming, where explicit instructions are given, ML systems improve performance by identifying patterns in large datasets and adapting as they receive more data.
AI is a broader concept, while ML is a technique used within AI to enable systems to learn from experience. An AI system that recognizes images can be programmed with specific rules, like identifying objects based on defined features. In contrast, an ML approach teaches the system using a large data set of labeled images, allowing it to learn how to identify objects without needing specific rules for each feature.
AI systems are designed to analyze data, adapt to new information, and improve over time, mimicking human cognitive functions like making decisions and understanding language. ML is a part of AI that allows machines to learn from data and improve over time without being programmed for each task. While AI includes techniques like machine learning, natural language processing, and computer vision, it refers to the overall technology that combines these methods to create intelligent systems.
Have the right resources in place
When beginning the AI and machine learning journey, many life science organizations struggle with limited internal expertise, resource constraints, or uncertainty around how to structure early-stage initiatives. This is where strategic outsourced workforce solutions come into play—and where Astrix provides critical value.
Astrix helps R&D labs and life sciences companies hit the ground running by offering highly specialized talent across data science, informatics, lab systems, and digital transformation. Our flexible staffing solutions allow organizations to:
- Rapidly scale up AI/ML capabilities
- Fill key skill gaps in data engineering, bioinformatics, and AI model development
- Gain immediate access to experts in LIMS, ELN, and scientific data management
- Minimize hiring overhead and internal disruption
- Accelerate time-to-value while reducing risk
Whether your organization is exploring pilot projects or building long-term AI infrastructure, Astrix enables you to align the right people to the right roles at the right time—helping you build momentum and ensure success from day one.
Why Partner with Astrix?
The path to AI data readiness in R&D is not just a technical challenge—it’s a strategic journey. It requires a thoughtful blend of data management, cutting-edge tools, cross-functional expertise, and flexible talent strategies.
Astrix is uniquely positioned to help life science organizations navigate this transformation. With decades of experience in scientific informatics and a deep bench of expert consultants, Astrix delivers customized workforce solutions, LIMS implementation expertise, and AI enablement strategies that meet your lab where it is—and take it where it needs to go.
Whether you’re just getting started or scaling enterprise-wide AI programs, Astrix can help you accelerate results, reduce complexity, and build a future-ready, data-driven R&D organization.
Contact us to learn more.
Case Study: LabWare Centralized Data Review for a Global Biopharmaceutical Company
Overview A global biopharmaceutical company specializing in discovery, development,... LEARN MOREWhite Paper: Managing Data Integrity in FDA-Regulated labs.
New White Paper LEARN MORELET´S GET STARTED
Contact us today and let’s begin working on a solution for your most complex strategy, technology and staffing challenges.
CONTACT US