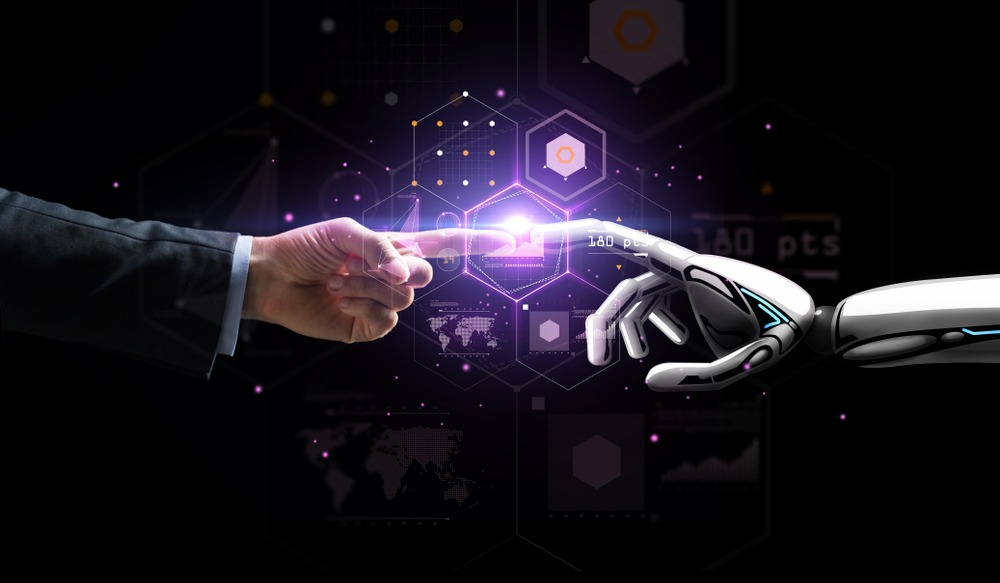
The Future of Artificial Intelligence and Machine Learning in Pharmacovigilance
Artificial Intelligence (“AI”) and Machine Learning (“ML”) are creating a buzz in the Pharmacovigilance (“PV”) space because of their transformative potential to provide new and interesting insights, by processing big data in a timely, accurate, and cost-efficient manner, and promises to enable product innovations to better protect patients and the world.
Defining Terms
Artificial Intelligence is a becoming challenging term to pin down because, increasingly, it’s applied across an exploding array of new approaches, applications, and products, but I like to think of it as, “The ability to train computers to do things that were, or are, being done by humans”.
To demystify this somewhat Orwellian description, let’s consider just two applications already in our daily life: cars with lane assist technology, and security measures involving facial recognition. AI is being employed to accelerate data-driven processes affecting our daily lives in ways that were nearly unthinkable (to anyone other than science fiction authors and NASA engineers) even a generation ago.
Pharmacovigilance can also benefit from AI—which promises to enhance human health via outcomes of product use—but it will require computers to deliver reliably better results than humans when using the same data. So, with this high of a bar, it makes sense that Artificial intelligence must rely on several subfields to actually facilitate true learning.
There are three subfields to know about right now:
- Machine Learning (“ML”) uses data, algorithms, and processes to mimic how humans learn without being explicitly programmed to do so. As the computer receives more data, it gradually improves its accuracy to predict outcomes. Although we won’t cover them in detail here, there are 3 types of ML that are rapidly emerging and developing:
- Supervised learning
- Unsupervised learning
- Reinforcement learning
- Deep Learning (“DL”) is a subset of ML that reaches a higher level of sophistication via focusing on pattern recognition in the same way that the human brain does. Speech and facial recognition are two examples of Deep Learning.
- Natural Language Processing (“NLP”) – is broadly defined as software that automatically manipulates natural language, like human speech and text. Language translation and analysis of unstructured data are two types of applications driven by NLP programs.
These three sub-areas of AI can—and often do— overlap as depicted in Fig 1
Given the critical nature of computations demanded of AI, and its sub-areas, there is intense focus on monitoring its performance in mission-critical applications. Professionals charged with developing and monitoring AI-supported programs use performance metrics to objectively assess AI processes for quality and consistency.
One popular performance metric used to evaluate how well ML is performing is the F1 score. The F1 score is a combination of precision and recall.
Precision is the number of True Positives divided by the number of Total Predicted Positives (True Positives + False Positives). Precision can be thought of as a measure of exactness. Therefore, low precision will indicate a large number of False Positives.
Recall is the number of True Positives divided by the number of real positive cases (True Positives + False Negatives). Recall can be thought of as a measure of completeness. Therefore, low recall indicates a large number of False Negatives.
The formula for the standard F1 Score is:
2*(Precision*Recall)
(Precision+Recall)
A strong PV system will be able to share or display its “confidence score” on each piece of extracted data, where it is located, and the algorithm(s) or approach(es) it used to obtain the confidence score. In addition, it is highly desirable for your PV system to be able to learn or improve as it receives more data and notifies someone within your organization when a new potential algorithm has been identified.
Future of AI and ML in Pharmacovigilance
AI is already having a profound impact on the performance of Pharmacovigilance programs, but we are still scratching the surface of AI’s potential role in PV program impact and efficiency. Here’s just a sample of the many areas in which AI can be applied:
- Reference Safety Information (“RSI”) Evaluate – Using current AI techniques, your system can read through any of the RSI literature (e.g., Investigative Brochure, Product Insert, Summary of Product Characteristics) to determine and extract the terms that should be used to assess expectedness. As the RSI literature changes, a company can rerun its algorithms and identify changes that need to be applied to its system.
- Causality Assessment – Some companies are already evaluating and piloting AI to identify the causality assessment. The problem that companies are seeing is that, in some cases, the F1 score is not high enough, simultaneously increasing manual reviews while decreasing confidence. As algorithms improve, and data increasingly runs through existing algorithms, this will continue to improve.
- Touchless Workflows – For certain types of cases with a high enough F1 and confidence score, it is feasible that a user would never have to see the case data. The system would automatically extract the data, process the case and, if required, report it to appropriate agencies. While some companies are piloting this for very specific low-risk non-serious cases. As AI technology improves and PV communities feel more confident with the technology, the volume of cases that would be “Touchless” will increase. This would have one of, if not the biggest, impacts on both the financials and time for processing data.
- Predictive Patient Risk – As regulatory agencies and companies receive more data AI can do one of the things it does best: analyze large amounts of data and identify significant patterns. AI could soon enable companies to identify which patients are at greatest risk for an adverse event to a prescribed medicine based on their genetic and biochemical characteristics.
Why it Matters to You
Even though AI is already having a significant impact on performance in the PV area, we have only begun to scratch the surface of AI’s potential impact on the effectiveness of PV programs.
In this blog we discussed:
- Key definitions of AI related terminology.
- A popular method to measure how well ML is performing.
- Where AI and ML are being used in life sciences.
- How AI and ML are impacting the evaluation of RSI Literature, Causality Assessments, Patient Risk.
To learn more about how AI and ML are impacting Pharmacovigilance you can download our white paper on this topic: Next Level Vigilance & Safety: Insights into the Transformative Potentials of AI & ML
About Astrix
Astrix is the unrivaled market-leader in creating & delivering innovative strategies, solutions, and people to the life science community. Through world class people, process, and technology, Astrix works with clients to fundamentally improve business & scientific outcomes and the quality of life everywhere. Founded by scientists to solve the unique challenges of the life science community, Astrix offers a growing array of strategic, technical, and staffing services designed to deliver value to clients across their organizations
Case Study: LabWare Centralized Data Review for a Global Biopharmaceutical Company
Overview A global biopharmaceutical company specializing in discovery, development,... LEARN MOREWhite Paper: Managing Data Integrity in FDA-Regulated labs.
New White Paper LEARN MORELET´S GET STARTED
Contact us today and let’s begin working on a solution for your most complex strategy, technology and staffing challenges.
CONTACT US