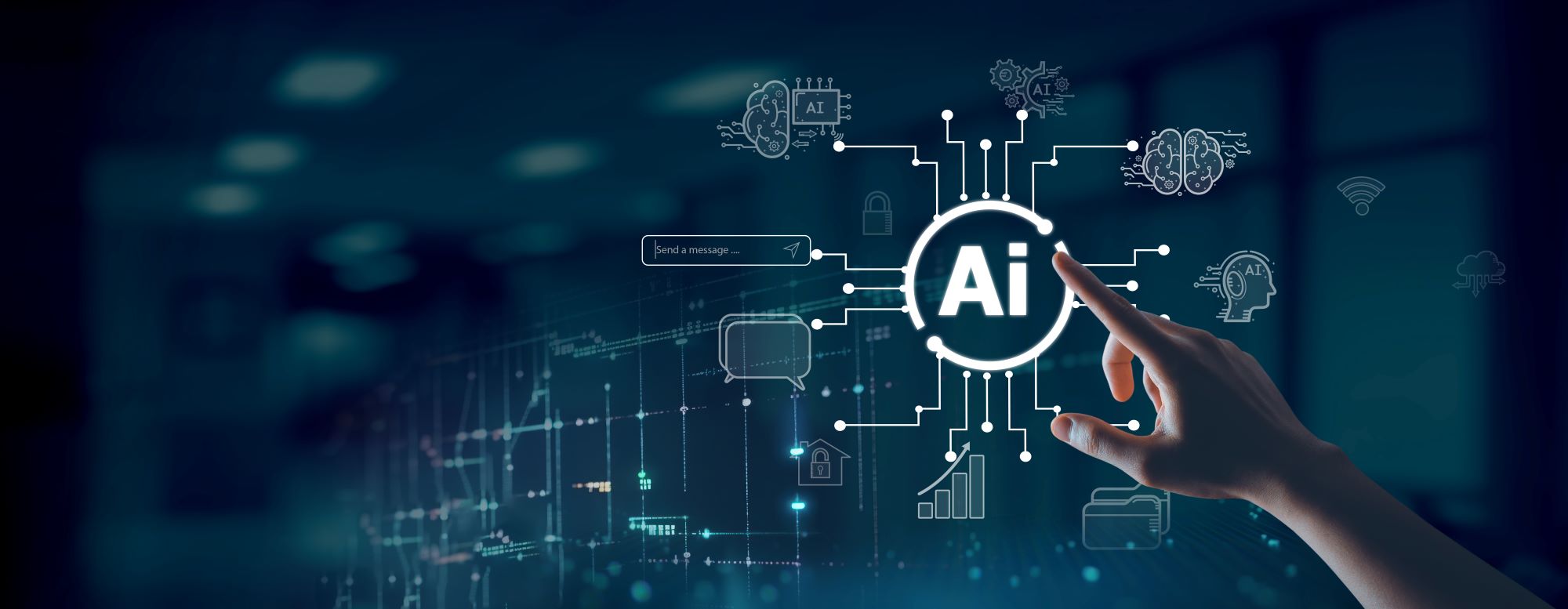
Harnessing AI and Machine Learning for Revolutionary Advances in Research and Scientific Organizations
The integration of Artificial Intelligence (AI) and Machine Learning (ML) in research and scientific organizations is revolutionizing the way data is analyzed and decisions are made. The recent webinar presented by Dave Dorsett, Principal Software & Systems Architect at Astrix, highlighted the current state of AI/ML, its applications, and practical steps for implementation. This article delves into the insights shared during the webinar, focusing on the strategic integration of these technologies in research and scientific environments.
Current State of AI/ML in Research
AI and ML in Research and Development Labs
The field of AI and ML has seen significant advancements, particularly in research and development (R&D) and quality control (QC) labs within the pharmaceutical, biotech, consumer packaged goods (CPG), and chemical industries. These advancements are primarily characterized by systems capable of generating outputs from inputs through cycles of active and reinforced learning. However, the scope remains narrow, and the self-learning capabilities required for general AI are still distant prospects.
Challenges and Opportunities
Despite the progress, there are challenges in achieving broader AI capabilities. The current systems are specialized and excel in specific tasks but lack the generalization necessary for broader applications. However, there are many opportunities for AI and ML in specialized research environments, with potential to significantly enhance efficiency and accuracy.
Applications of AI in Life Sciences
1. Discovery
Target Identification and Candidate Screening
- AI models help in identifying potential drug targets by analyzing large datasets for patterns and anomalies. Machine learning algorithms can screen candidates more efficiently, reducing the time and cost associated with drug discovery.
De Novo Design and Discovery
- AI aids in the de novo design of molecules, predicting their properties and potential efficacy. This accelerates the discovery process and enhances the precision of research outcomes.
2. Non-Clinical Research
In-vivo Simulation and Integrated PK/PD Modeling
- AI-driven simulations provide insights into how drugs interact within living organisms, helping researchers predict pharmacokinetics (PK) and pharmacodynamics (PD). These models improve the understanding of drug behavior and efficacy.
3. Clinical, Development, and Manufacturing
Process Design Optimization and Control
- In the development and manufacturing phases, AI optimizes process design, ensuring maximum performance and safety. AI systems monitor critical parameters, enabling dynamic adjustments and continuous quality improvement.
4. For General Purposes
Data Governance, Workflow, Data Tagging, and Classification
- AI enhances data governance by automating data tagging, harmonization, and classification. This improves data findability and usability, supporting efficient workflows and better decision-making.
How to Start Implementing AI/ML
1. Initiate and Plan the Project
- Identify the core and extended teams and involve key stakeholders. Develop a comprehensive project plan and communication strategy to ensure alignment and understanding.
2. Define Future State Strategy and Roadmap
- Collaborate with subject matter experts (SMEs) and leadership to refine the strategy. Prioritize projects, systems, and workstreams based on organizational goals and capabilities.
3. Develop Conceptual Data and System Reference Architecture
- Create a reference architecture that aligns with business needs and data capabilities. This architecture serves as a strategic guide for future state development.
4. Solicit and Review Stakeholder Feedback
- Engage with stakeholders to identify current challenges and future aspirations. Develop a capabilities assessment to understand the existing ecosystem and prioritize needs.
5. Confirm Business and Data Capabilities
- Align stakeholders on business drivers, operational and technical needs, and clearly articulate the vision and objectives.
Ensuring Data Quality
Data quality is paramount in AI/ML applications. Poor input data leads to poor outputs, making it crucial to have digitalized, harmonized, and mature data. This includes ensuring data provenance, maintaining lineage, and managing changes transparently.
Organizational Aspects of AI/ML Implementation
Effective AI/ML implementation requires balancing technical achievements with scientific objectives. Organizations can adopt a decentralized model where business units define and assess applications, using centralized technology as needed. This approach ensures that AI/ML efforts are science-driven and build value rather than just technology.
General-Purpose Applications
AI and ML can be applied to various general-purpose tasks such as data classification, system integration, and improving operational systems through automation. Viewing AI/ML models as tools for scientists and SMEs helps maintain rational expectations and addresses transparency challenges.
Watchpoints in AI and Machine Learning Implementation
AI/ML models often produce outputs with high confidence, even when incorrect. It is crucial to have mechanisms to validate outputs and ensure they are reliable. This includes monitoring model quality, validating human factors, and periodically retesting models.
Validation Framework for AI Systems
Validation of AI systems involve a structured approach, ranging from classical non-AI validation to advanced autonomous learning systems. Each level requires specific validation steps to ensure model quality, data integrity, and compliance with regulatory standards.
Common Questions About AI/ML in Life Sciences
What are the key steps to start implementing AI/ML in research organizations?
- Starting with AI/ML implementation involves initiating and planning the project, defining a future state strategy, developing conceptual data architecture, and ensuring data quality. Engaging stakeholders and confirming business and data capabilities are also crucial steps
How does AI improve drug discovery?
- AI enhances drug discovery by identifying potential targets, screening candidates, and predicting the properties of new molecules. This accelerates the discovery process and improves precision.
What role does data quality play in AI/ML applications?
- Data quality is critical as AI and Machine Learning models rely on accurate and harmonized data. Poor data quality leads to unreliable outputs, making it essential to ensure digitalized, structurally harmonized, and mature data.
How can organizations balance technical and scientific goals in AI/ML implementation?
- Organizations can adopt a decentralized model where business units define and assess applications, using centralized technology as needed. This ensures that AI/ML efforts are science-driven and build value rather than just technology.
What are some general-purpose applications of AI in research?
- AI can be used for data classification, system integration, and improving operational systems through automation. These applications enhance data governance, workflow enablement, and decision-making.
What are the validation requirements for AI systems?
- Validation of AI and Machine Learning systems involve structured steps ranging from classical non-AI validation to advanced autonomous learning systems. Each level requires specific validation to ensure model quality, data integrity, and regulatory compliance.
Conclusion
The integration of AI and Machine Learning in research and scientific organizations holds immense potential for enhancing efficiency, accuracy, and innovation. By following a structured implementation strategy, ensuring data quality, and addressing organizational and validation aspects, organizations can successfully harness the power of AI and Machine Learning. Dave Dorsett’s insights provide a valuable roadmap for navigating this complex yet rewarding journey.
How Astrix Can Help
For over 28 years, Astrix has been at the forefront of digital transformation in the life sciences and science-based sectors. Our unique blend of specialized strategic, technical services, streamlined processes, and technology-agnostic approach has made us a trusted partner for life science organizations seeking to enhance their operational success. Through innovative solutions, expert consulting, and robust support, Astrix is dedicated to driving the advancement and efficiency of our clients.
Ready to transform your organization’s digital journey? Contact us now to learn more about our services and how we can help your business thrive in the digital age.
Case Study: LabWare Centralized Data Review for a Global Biopharmaceutical Company
Overview A global biopharmaceutical company specializing in discovery, development,... LEARN MOREWhite Paper: Managing Data Integrity in FDA-Regulated labs.
New White Paper LEARN MORELET´S GET STARTED
Contact us today and let’s begin working on a solution for your most complex strategy, technology and staffing challenges.
CONTACT US