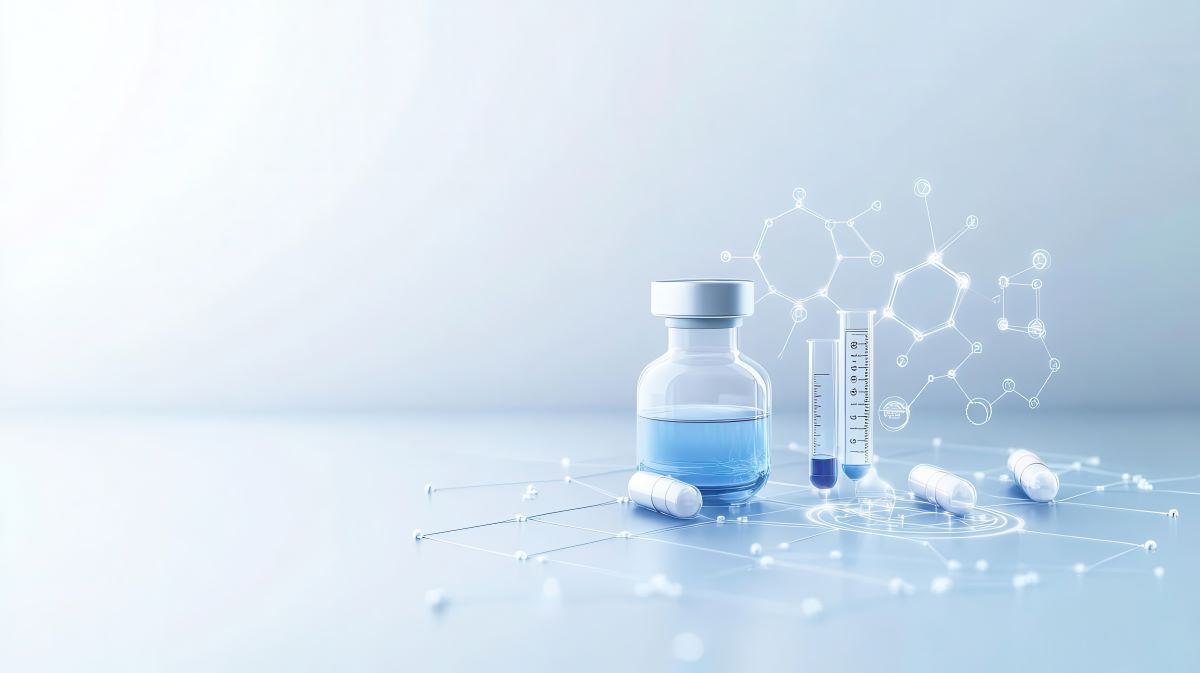
AI in Drug Repurposing: Finding New Uses for Existing Medications
The field of medicine has seen an explosion of technological advancements in recent years, and artificial intelligence (AI) is at the forefront of this revolution. One of the most promising areas where AI is making a significant impact is in drug repurposing. This practice involves identifying new therapeutic applications for existing drugs, which can dramatically accelerate the development of effective treatments and reduce the costs associated with drug discovery (Ekins et al., 2019).
Why Drug Repurposing?
Traditional drug development is a long, complex, and costly process, often taking 10–15 years and billions of dollars to bring a new drug from discovery to market (Ashburn & Thor, 2004). Drug repurposing, however, leverages medications that have already been tested for safety, allowing researchers to bypass some of the most time-consuming and expensive early stages of research. This not only saves time but also ensures treatments are made available to patients faster, potentially addressing unmet medical needs sooner (Pushpakom et al., 2019).
The Role of AI in Drug Repurposing
AI is transforming drug repurposing by analyzing vast amounts of biomedical data to identify potential new uses for existing drugs. Machine learning algorithms are capable of sifting through clinical trial results, scientific literature, genetic data, and patient records to reveal hidden connections between drugs and diseases that may not be immediately apparent to human researchers (Bertolini et al., 2021).
Applications of AI in Drug Repurposing:
- Data Mining and Pattern Recognition: AI systems can scan millions of scientific papers and clinical trial reports to identify patterns indicating that a drug approved for one condition might be effective for another (Shameer et al., 2018).
- Predictive Modeling: Machine learning models can predict how drugs interact with different biological pathways, enabling researchers to focus on promising candidates for repurposing (Zhang et al., 2017).
- Real-World Evidence Analysis: AI can analyze electronic health records (EHRs) and real-world patient data to observe how drugs used off-label affect various conditions, identifying new repurposing opportunities (Wang & Liu, 2020).
- Genomic Analysis: By analyzing genomic and proteomic data, AI algorithms can suggest how a drug might work on molecular targets linked to different diseases, facilitating targeted repurposing efforts (Chandak et al., 2021).
Success Stories of AI in Drug Repurposing
A notable success story is the use of AI to identify drugs for treating rare diseases. These conditions often do not attract the funding necessary for new drug development, but AI has helped repurpose existing treatments effectively. For example, drugs originally designed for cancer treatments have been repurposed to manage rare metabolic disorders due to the shared molecular pathways that AI models identified.
During the COVID-19 pandemic, AI also played a crucial role in rapidly identifying existing drugs that could be repurposed to manage the disease. Algorithms combed through databases to suggest antivirals and anti-inflammatory medications that could reduce severe symptoms and improve patient outcomes.
Challenges in AI-Driven Drug Repurposing
Despite its promise, AI in drug repurposing is not without challenges:
- Data Quality and Integration: AI models depend on the quality and integration of data from multiple sources. Inconsistent or incomplete data can lead to inaccuracies.
- Complex Biology: Biological systems are incredibly complex, and the mechanisms of action for drugs can be multifaceted, making predictions challenging even for sophisticated algorithms.
- Regulatory Hurdles: Even repurposed drugs must undergo clinical trials to validate their efficacy and safety for new indications, which can still take years and significant investment.
The Future of AI in Drug Repurposing
As AI technology evolves, it will become an even more powerful tool for drug repurposing. Integrating AI with advanced computational techniques such as network biology and omics sciences will deepen our understanding of disease mechanisms and drug interactions. This, in turn, will enhance AI’s predictive power, leading to faster and more accurate identification of potential treatments.
As more health data becomes digitized and accessible, AI will be able to analyze larger and more diverse datasets, making it more adept at finding novel drug applications that benefit broader patient populations.
Conclusion
AI’s role in drug repurposing holds immense potential to revolutionize the pharmaceutical industry by unlocking new uses for existing medications. With the capability to save time, reduce costs, and accelerate treatment availability, AI-driven drug repurposing is set to become an essential tool in modern medicine. As challenges in data integration and regulatory processes are addressed, the future will likely see an increasing number of success stories where AI helps bring life-changing treatments to patients faster than ever before.
About Astrix
Astrix is a strategic partner that helps companies in the life sciences harness AI capabilities by integrating AI into their workflows through consultative and staffing solutions. For over 28 years, Astrix has supported organizations by providing the talent needed to drive forward innovation and meet operational goals effectively.
Learn more about our consulting services.
Learn more about our staffing solutions.
References
- Ashburn, T. T., & Thor, K. B. (2004). Drug repurposing: identifying and developing new uses for existing drugs. Nature Reviews Drug Discovery, 3(8), 673-683.
- Bertolini, F., Sukhatme, V. P., & Bouche, G. (2021). Drug repurposing in oncology—patient and health systems opportunities. Nature Reviews Clinical Oncology, 18(12), 733-744.
- Chandak, M., Wang, D., & Zhang, Y. (2021). AI and Genomic Analysis in Drug Repurposing. Trends in Pharmacological Sciences, 42(6), 425-440.
- Ekins, S., Puhl, A. C., Zorn, K. M., Lane, T. R., Russo, D. P., Klein, J. J., & Siqueira-Neto, J. L. (2019). Exploiting machine learning for end-to-end drug discovery and development. Nature Reviews Drug Discovery, 18(6), 463-477.
- Pushpakom, S., Iorio, F., Eyers, P. A., Escott, K. J., Hopper, S., Wells, A., … & Pirmohamed, M. (2019). Drug repurposing: progress, challenges, and recommendations. Nature Reviews Drug Discovery, 18(1), 41-58.
- Richardson, P., Griffin, I., Tucker, C., Smith, D., Oechsle, O., Phelan, A., … & Stebbing, J. (2020). Baricitinib as potential treatment for 2019-nCoV acute respiratory disease. The Lancet, 395(10223), e30-e31.
- Shameer, K., Brown, A. J., Liston, D. R., & Johnson, K. W. (2018). AI-driven clinical trial matching: how AI can improve clinical trial recruitment and outcomes. Nature Reviews Drug Discovery, 17(9), 667-668.
- Wang, Y., & Liu, Z. (2020). AI in Drug Repurposing: Challenges and Opportunities. Pharmaceutical Research, 37(8), 1-15.
- Zhang, M., Xiao, S., & Shen, W. (2017). Machine learning approaches in drug repurposing: Current applications and future directions. Computational and Structural Biotechnology Journal, 15, 449-456.
Case Study: LabWare Centralized Data Review for a Global Biopharmaceutical Company
Overview A global biopharmaceutical company specializing in discovery, development,... LEARN MOREWhite Paper: Managing Data Integrity in FDA-Regulated labs.
New White Paper LEARN MORELET´S GET STARTED
Contact us today and let’s begin working on a solution for your most complex strategy, technology and staffing challenges.
CONTACT US